Quantitative Research
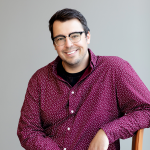
11/10/21
Examining The New Way The 2020 Census Asked About Race
We explore what this change means for data collection and what other adjustments we might expect in the future.
By Jim PripusichRead More
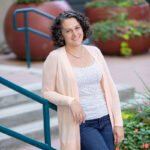
11/2/21
A Look Into Other North American Census Questionnaires
While we have all filled out the US Census, have you ever considered how other North American nations handle their census?
By Annie TheodoropoulosRead More
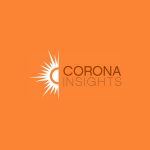
7/30/20
What is data storytelling?
At Corona, we are constantly trying to think of better ways to present data so that it is easy to use. We recently gave a presentation to our friends at Denver Data Storytellers about blended storytelling. Below is a summary of what we discussed.
By Caitlin McAteerRead More
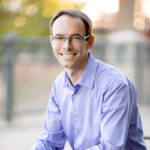
7/23/20
What is a Push-to-Web Survey?
Push-to-web survey approaches are growing in popularity. But what exactly is a push-to-web survey, and what are best practices?
By Matt BruceRead More
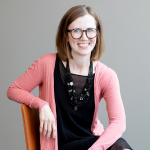
12/18/17
How to measure what people want
Recently after an interview for a project, some us at Corona had a discussion about whether or not it would be useful to use a survey for the project. Like a lot of projects, this potential client was interested in what new changes the public might want in their organization. And at first, this seems […]
By Kate DarwentRead More
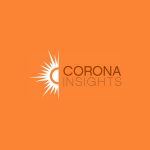
11/15/17
Breaking down the wall between quant and qual
Recently we had a project involving a large survey with numerous open-end questions. Taking the divide and conquer approach, it was all hands-on deck to quickly code the thousands of responses. As a qualitative researcher, coding survey responses can feel like a foreign process and I often found myself overthinking both my codes and the […]
By Molly HaganRead More
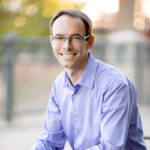
11/5/17
The Four Cornerstones of Survey Measurement: Part 2
Part Two: Reliability and Validity The first blog in this series argued that precision, accuracy, reliability, and validity are key indicators of good survey measurement. It described precision and accuracy and how the researcher aims to balance the two based on the research goals and desired outcome. This second blog will explore reliability and validity. […]
By Matt BruceRead More
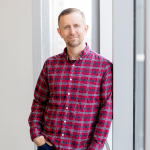
11/3/17
Keeping it constant: 3 things to keep in mind with your trackers
When conducting a program evaluation or customer tracker (e.g., brand, satisfaction, etc.), we are often collecting input at two different points in time and then measuring the difference. While the concept is straightforward, the challenge is keeping everything as consistent as possible so we can say that the actual change is NOT a result of […]
By David KennedyRead More
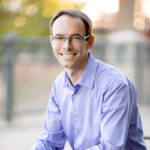
10/30/17
The Four Cornerstones of Survey Measurement: Part 1
Part One: Precision and Accuracy Years ago, I worked in an environmental lab where I measured the amount of silt in water samples by forcing the water through a filter, drying the filters in an oven, then weighing the filters on a calibrated scale. I followed very specific procedures to ensure the results were precise, […]
By Matt BruceRead More
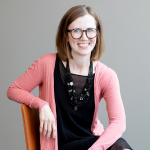
5/31/17
Thinking strategically about benchmarks
When our clients are thinking about data that they would like to collect to answer a question, we sometimes are asked about external benchmarking data. Basically, when you benchmark your data, you generally are asking how you compare to other organizations or competitors. While external benchmarks can be useful, there are a couple of points […]
By Kate DarwentRead More