There is more to a quality survey than margin of error
5/20/16 / Matt Herndon
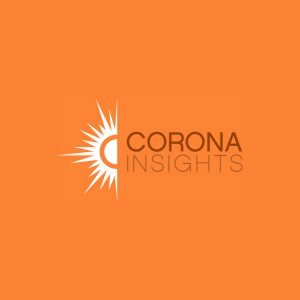
One of the areas in which Corona excels is helping our clients who aren’t “research experts” to understand how to do research in a way that will yield high-quality, reliable results. One of the questions we are frequently asked is how many completed surveys are necessary to ensure a “good survey.” While the number of surveys definitely has an impact on data quality, the real answer is that there are many things beyond sample size that you have to keep in mind in order to ensure your results are reliable. Here is an overview of four common types of errors you can make in survey research.
Sampling Error
Sampling error is the one type of error that can be easily summarized with a number. Because of this, many tend to think of it as the main way of reporting a survey’s quality. Sampling error refers to the “margin of error” of the results of a survey caused by the fact that you didn’t survey everyone in the population you are surveying – only a sample. The “error” occurs when you draw a conclusion based on a survey result that may have been different if you’d conducted a larger survey to gather a wider variety of opinions. As an example, imagine that you wanted to conduct a survey of people at a concert about their reasons for attending. In an extreme case, you could collect 10 random responses to your question and draw conclusions about the population from that, but chances are the next 10 people you hear from might have very different opinions. As you collect more and more surveys, this becomes less of an issue.
It’s important to keep in mind, however, that the calculations for sampling error assume that 1) your sample was random (see coverage error below) and 2) everyone you chose for the survey ended up responding to the survey (see non-response error below) – neither of which are true a lot of time. Because of that, it is important to realize that any margin of error calculation is likely only telling you part of the story about a survey’s quality.
Still, it is certainly true that (when obtained properly), larger sample sizes tend to result in more reliable results. Generally speaking, a sample of 100 responses will give you a general feel for your population’s opinions (with margins of error around ±10 percent), 400 responses will give you reasonably reliable results (with margins of error around ±5 percent), and larger samples will allow you to examine the opinions of smaller segments of your audience, so obtaining a large sample size will always be a key consideration in survey research.
Measurement Error
Measurement error is one of the most difficult types of errors to identify and is probably the most common mistake made by amateur survey researchers. Measurement error occurs when respondents don’t understand how to properly respond to a question due to the way it’s worded or the answer options you’ve provided. For example, if you were to ask concert goers how long it took them to get to the venue that night, you might get a set of answers that look reasonable, but what if some picked up their friends on the way? What if some went to dinner before the concert? Similarly, if you asked whether they came to the concert because of the band or because they like the venue, you might conclude that a majority came for the band when the real reason was that many just wanted to hang out with their friends.
So how do you protect against this? Whenever possible, it’s important to test your survey – even if it’s just with a friend or coworker who is not involved in the research. Have them take the survey, then have them talk you through how they interpreted each question and how they decided which answer fit best. Then, if necessary, make changes to your survey in any areas that were unclear.
Coverage Error
Once you have a well-designed set of survey questions developed, the next step is to determine how you are going to get people to take your survey. It might be tempting to just put a link on the concert venue’s Facebook and Twitter pages to ask people their opinions, but the results of such a survey likely wouldn’t reflect the opinions of all concert goers because it’s unlikely that all of them use social media. If you were to do a survey in this fashion, you might find that attendees tended to be very tech savvy and open to new ideas and approaches, when the reality was that those just happen to be characteristics of people who use social media. The results of your survey might be skewed because you didn’t “cover” everyone in the audience (not to mention the possible issue of some taking the survey that didn’t actually attend the concert).
In order to ensure your survey is as high quality as possible, look for ways to ensure that everyone you are trying to represent is included in your sampling frame (even if you randomly choose a subset to actually receive an invitation). If it’s truly not possible to do so, be sure to at least keep the potential bias of your respondent pool in mind as you interpret the results.
Non-response Error
As the final type of common error, non-response error is caused by the fact that, no matter how well you have designed and implemented your survey, there are a lot of people out there who simply aren’t going to respond. Similar to coverage error discussed previously, non-response can cause you to draw conclusions from your survey’s results that may not be reflective of the entire population you are studying. For example, many concert goers wouldn’t want to be bothered to take a survey, so the results you get would likely only be representative of a type of person who either 1) didn’t value their time as highly or 2) was particularly interested in the survey topic.
Unfortunately, non-response error is extremely difficult to eliminate entirely and will be a concern with just about any survey. The most common approach is to try and boost your response rate as much as possible through a combination of frequent reminders, incentives, and appealing to respondents’ desire to be helpful in your messaging about the study, but even the best surveys typically only achieve response rates of 30-40 percent. If budget is no issue, perhaps the best solution is to conduct follow-up research with those that didn’t originally respond, but even then, there will always be some who simply refuse to participate.
~
When it comes down to it, there is no such thing as a perfect survey, so any study will necessarily need to balance data quality with your timeline and budget. Many of Corona’s internal debates involve discussing ways to reduce these errors as effectively as possible for our clients, and we are always happy to discuss various tradeoffs in approaches and how they will impact data quality. Regardless, we hope that the next time you see a headline about a survey with a margin of error used to represent its quality, you will keep in mind that there is a lot more to determining the quality of a survey than that one number.