Build Your Team’s Data Literacy
12/10/23 / Paul Collier
You likely interact with data every day in making decisions for your organization, programs, and clients. While you understand the importance of data in your work, you are not a statistician or researcher. Maybe you’re just a little rusty on your statistics terms. Or, maybe you feel as if the “data wizard” tasked with analyzing data at your office holds a magic wand that you’ll just never understand.
I’ve found that these are common feelings among nonprofit staff, managers, and leaders. However, data doesn’t have to be intimidating. After working with nonprofits and social enterprises of all sizes, I’ve seen that even the smallest and most budget-constrained organizations can use data in useful ways, like:
- Making better decisions on where to spend their time
- Building trusting relationships with partners and funders
- Adjusting their services to better meet their client’s needs
I’ve learned that there are simple things that you and your team can do to build your collective comfort with data, and I want to share these with you. This is the first in a three-part series about practical steps you can take to maximize your nonprofit’s existing data assets. This series is based on my experience helping over three dozen direct service nonprofits with less than fifty staff members leverage their data more effectively. In this post, we will dive into the foundation of data use: data literacy.
“The house that data built”
A helpful metaphor for your nonprofit is “the house that data built.” This particular house (left) was 3-D printed and can be constructed in a day to provide shelter in disaster zones. Your organization’s “house that data built” will take longer to put together, but like this house, it will have a predictable set of components and will come together in a structured way.
In this “house,” individual data literacy is the foundation. Data literacy – a common language for understanding and using data – enables the structure to stand. The structure of an organization is its culture – the habits for working together and using data to support collaboration. Within these walls are windows, some of which are specifically related to the data you collect and others that are related to how you use data. This culminates in the roof of your “house that data built” – scalable impact. Once individuals in your organization have a common language for data use and shared habits for using data, your organization can demonstrate that it can (and should) grow.
The Foundation: Building Data Literacy
Data literacy is the ability to think critically about data, and transform data into products that will help other people learn. This is true no matter what your role is in the organization. You might be a data author – someone who creates analyses & reports. This is the role that I often play. Or, you may be a data consumer – someone who reads reports created by others – such as an Executive Director or Program Manager. You also could be a data agnostic – someone who doesn’t deal with data often – though it’s becoming more and more rare that someone doesn’t need to deal with data as part of their job.
Knowledge + Terms
Individual knowledge is a prerequisite to using data in service of your organization’s mission. The foundation of individual knowledge is understanding data terms. For the average data consumer or data agnostic, memorizing data terms isn’t important; but being familiar with key terms is. Some of the most important data terms I find myself explaining time and again include:
- Data types: Each variable in a dataset has a type, and different data types lend themselves to different kinds of analysis. Common types of data you may encounter range from qualitative data to quantitative data. These two termsare not binary; instead, they are ends of a spectrum. Different types lend themselves to different analysis approaches. For example, there are different statistical tests for quantitative data that have an order (e.g., numeric rankings) than data that are unordered (e.g., demographic categories). Qualitative data, like pictures or quotes, can often tell a compelling story, but a small number of these may not adequately describe the range of experiences for everyone your organization serves.
- Data cleaning: This is the process of reviewing and editing or omitting raw data received to ensure data used in an analysis is as accurate as possible. Messy data can be caused by system failures, human error, or differences in data collection practices between individuals and over time. Aspects of data cleaning include: editing existing but messy data; identifying missing values on existing records; removing duplicate records; and identifying records missing entirely. Data cleaning often takes the largest amount of time of an analysis project (25-50% of the total project time).
- Distribution: A list of all values for a variable and the frequency at which those values occur in a data set. A common distribution is the normal distribution or bell curve, where most data points cluster around the average value. It’s important to remember that it’s not just the average, median or mean that matters (where the data clusters) but also what the range is of the data.
- Correlations vs. causation: This is an important distinction when interpreting results, and one of the central challenges of evaluation! Correlation means that a relationship exists between two or more variables. Causation means that a causal relationship exists between two or more variables. Most statistical tests looking at data can estimate correlation, but an experiment with some kind of control group is often needed to statistically prove out a causal relationship. For most of us in the nonprofit sector, we are looking at correlations in our data and using them to illustrate our impact on our clients, mission, and society. That’s often totally appropriate. But as your organization grows, some funders or partners may expect to see data that proves causation – your program (not something else) causes important changes for those you serve.
Being a good data consumer
A good data consumer asks three questions: Where does this data come from; what can I learn from it; and what can I do with it.
1) Where does this data come from?
In asking where data comes from, I like to use the example of different sources of news media. Which do you think is more trustworthy, a tabloid daily free newspaper, an airline monthly in-flight magazine, or The Economist. In thinking about the purpose of each of these publications, there are differences in: the audience, resources, nature of the stories, type of writing, and level of research undertaken to publish them. Data is no different. You would still want to know a few important things about your source:
- What are the strengths and weaknesses of this source?
- What real life behavior do the data represent?
- What information is emphasized?
- What level of granularity is shown?
- What is the scope of the data (e.g., time, program, or geography)
2) What can I learn from it?
Remember that data analysis is both an art and a science.One of the first things to do when looking at data is to compare your results to your expectations. Consider ahead of time what results would make you pleased and what results would make you concerned. Next, look for patterns or relationships between different pieces of data you’re shown and trends over time. Are you seeing any positive, negative or neutral relationships? What effect does seasonality or time have on the results you’re looking at? Lastly, take a good look at outliers because they can point to a data quality concern or a unique situation that might shed light on the rest of your results.
3) What can I do with it?
Thinking about what you can do with the data should be a group process, involving more than one person in your organization. A framework of thinking that I use and recommend to my clients is an emergent learning map (EL map). While this came from the US Army, it has been popularized in other sectors as a way to make decisions based on data in a group setting. EL maps organize a data review session around one important question. The discussion progresses from step one (grounding truth) to discussing insights, hypotheses, and opportunities for action.
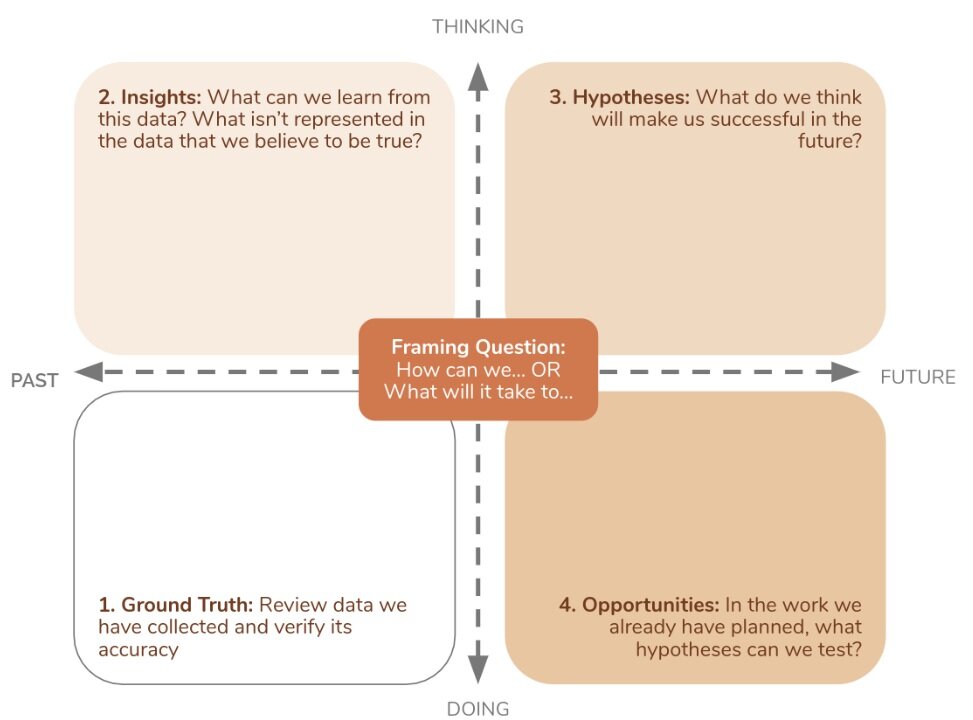
Understanding what data analysis really means
Finally, it’s important that you and your team know what goes into a data analysis cycle. Even if you’re not doing the analysis, having this knowledge will build your ability to work effectively with an analyst. Data analysis takes time and is overall an iterative process. While the particulars of an analysis process vary, data analysis tends to follow six common stages.
- Define or redefine the question you are asking
- Assess the structure and quality of the data–its strengths and weaknesses.
- Transform the dataset. Often there will be results that need to be structured in a different way to pull the right chart or do the proper statistical tests. This step also includes data cleaning (dealing with duplicates, missing values, etc.).
- Analyze visually by creating the graphs or charts to represent the datas spread and interrelationships.
- Analyze statistically- depending on the question, the analyst will see if the data varies in statistically meaningful ways between different groups or over time
- Draft the results in a format appropriate for the audience and share the results. Often times the initial results will prompt more questions, and the process may repeat itself.
As you can tell, data analysis takes some work! So the next time you read a report or request analysis help, you can have some empathy for those who are doing the hard work behind the scenes.
Conclusion
Individual data literacy is an important professional skill for anyone, and its also the foundation for organizational data use. Once you and your team members have a common language around data and understand what it takes to be a good data consumer, you can move on to establishing habits for data collection and analysis that help your team learn. Investing in your organization’s data infrastructure will ultimately help you and your team make better decisions around how to use your resources, communicate your impact to funders and partners, and improve your services.
Resource: Want to share this with your team? Feel free to download my Data Literacy Handout, which covers everything we discussed in this blog and more. I welcome you to edit this to include terms and concepts that are important for your team!
If you would like to learn more about how Coeffect can help your organization build its data literacy, schedule time with Paul.
View the second post in this series here.
This post by Paul originally appeared on the Coeffect.co website.