Can we use AI for qualitative research?
5/9/23 / Annie Theodoropoulos
With the recent launch of ChatGPT, Bard, and other chatbots, Artificial Intelligence has become a popular topic for the seemingly infinite opportunities it opens, as well as the kinds of disruptions it could potentially stir up.
Some pundits have troublingly alleged that AI could obviate labor that was once strictly considered the purview of an analytical human mind. Fears surrounding encroaching AI range from higher education and the assumption that students will simply have chatbots produce the kind of “thinking” work that would otherwise tell a professor whether or not they absorb material, to AI taking over the white-collar work of paralegals, physician assistants, etc., to even qualitative research fields whose bread and butter lies in uncovering meaning through the subtleties of human communication.
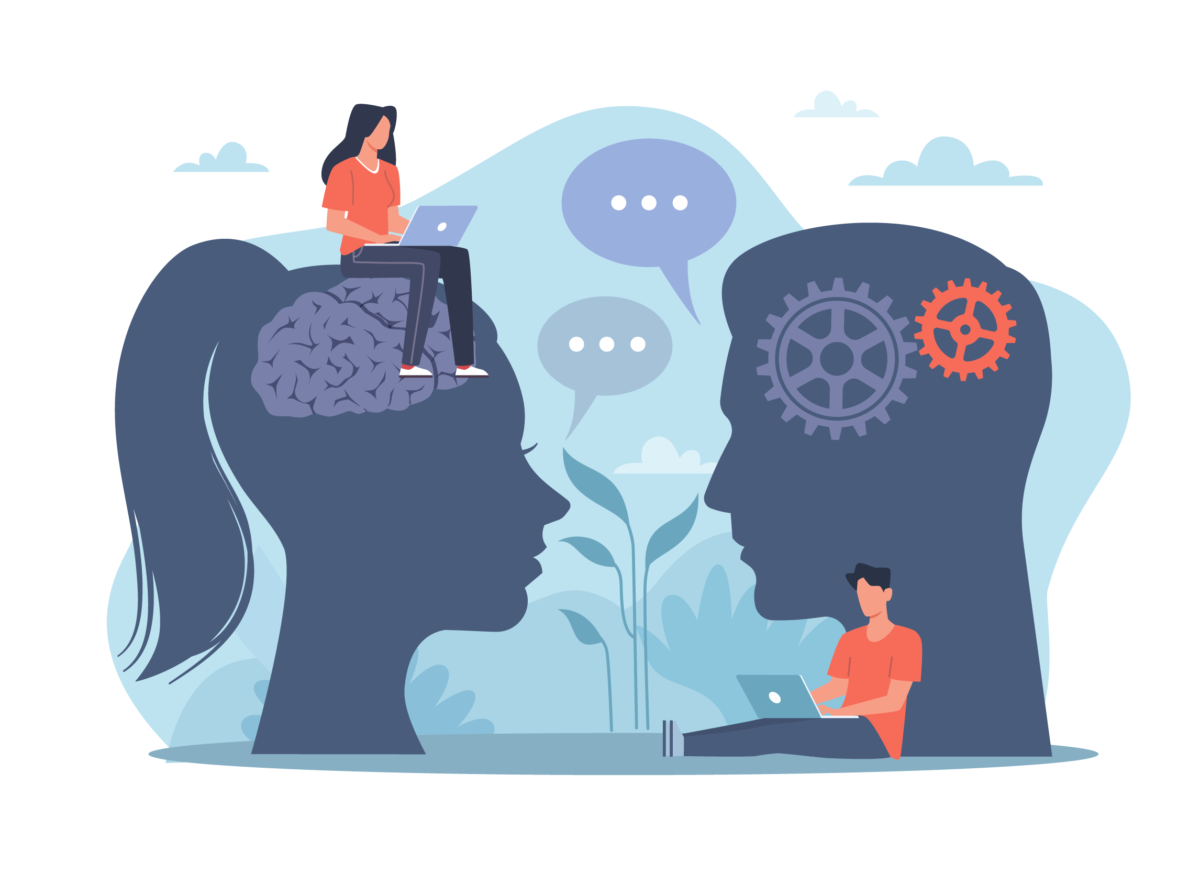
Are these fears legitimate? Will we have to adapt our systems to incorporate AI before AI simply takes over our positions? Many seem to think that the answer probably lies somewhere in the middle, where researchers become more adept at asking AI the right sorts of questions to fully leverage the mass data-scouring power it offers.
So, how can we use the new AI tools like ChatGPT now? What could they offer a qualitative researcher who has yet to go hook, line, and sinker for a full AI-driven analytical package? And, yes, these already exist and are being promoted at conferences worldwide!
AI IN RESEARCH DESIGN
Before we get ahead of ourselves and ask how an AI can navigate an entire analysis of qualitative data, let’s start more simply and ask what current AI can do to help us develop, say, an interview guide. For example, you consult ChatGPT with the premise that you are an interviewer looking to recruit undocumented migrant laborers and you need an appropriate interview guide that asks about their experiences. You will first receive a generic warning that using an AI language model for writing your questions raises ethnical concerns as it could “potentially put the workers in a vulnerable position.” That is an important note that requires the researcher to understand the scope of what that means, despite the banality of the statement.
Nevertheless, ChatGPT produces a list of questions:

At first glance, maybe these seem fine—there is something there to work with in terms of asking about personal work experience, challenges, positives. These questions feel especially geared toward the perspective of the interviewer, and that makes sense because what ChatGPT is trained on are digital documents available online from all kinds of private, academic, or non-profit organizations based on their research needs. We quickly run into trouble with unwieldy phrases like “conditions of employment” and “improving the lives of farmworkers.” A researcher would likely reject these questions because of the overly formal language and the enormous scope implied in connecting one person’s experience to the entirety of the plight of “farmworkers.” These questions should raise a red flag (e.g., Would interviewees see themselves in such a monolithic category as ‘farmworker’? Are other identities excluded by this framing? etc.).
LANGUAGE MODEL VS. LINGUISTIC MENTAL MODEL
This brings us back to the original caveat statement from ChatGPT: it is a language model, ultimately derived from a mathematical model trained on certain large sets of unknown inputs—and its algorithms for output generation are a proprietary “black box” that we cannot know. To put it simply, ChatGPT (and other AI bots) approximate the next word in a series of words that are deemed most likely to appear as a sentence. The algorithm is trained on vast inputs of sentences, which were created by people from a variety of contexts. What seems like the likely phrasing to an algorithm may not make much sense to a living, breathing person.
If you were to ask a human to create a list of interview questions, the human relies on a linguistic mental model of the type of perceived interviewee. This linguistic mental model is contextual and dynamic, informed through lived experience, imagination, and empathy. That is, when researchers approach and write questions, we intrinsically apply our mental model—our imagination based on experience—of how a question will play out with an interlocutor. This is very much determined by how we see the interlocutor, how we see ourselves, and how we see the interaction occur. This “seeing” happens in our mind’s eye based on the myriad experiences we’ve had before with other interlocutors, and informs the boundaries of our imagination, or how we perceive the context and the next steps of an interaction possible therein. To imagine how a line of questioning might go, we ask ourselves questions that experience has shown us to be relevant: are we in a public or private place, how does our language and self-presentation impact how the interlocutor approaches us, are we talking to someone who thinks of themselves as unique or representative, new or experienced? etc. etc. Our empathy, or ability to hold our interlocutor in our mind’s eye and see through their vantage point, informs how we triage these questions and weigh out the most important considerations first.
FINAL THOUGHTS
By all means, let’s get comfortable with AI and learn how to ask it all kinds of questions. However, it is crucial to take its own warnings into account. It seems that for now, AI is best utilized when what we need is a rough draft to get started. However, it cannot yet generate the kind of imaginative and empathetic work required to “think” for us.